Driving Real-Time Data Solutions: Insights from Uber's Na Yang
As the organizers of Flink Forward, at Ververica we take great pride in bringing together the Apache Flink® and streaming data communities. Every year, we appoint a Program Chair responsible for curating a diverse Program Committee. These committee members hail from diverse industries, each possessing extensive expertise in Apache Flink and streaming data technologies. They carefully evaluate talk submissions from the community to shape the Flink Forward program.
We’re proud to welcome Na Yang, Engineering Manager of Uber's Flink Platform team, as one of this year’s Flink Forward Program Committee members. Let's delve into her perspectives on the evolving landscape of real-time data analytics and the pivotal role Apache Flink plays within it.
Your experience spans across various tech giants like MapR, PayPal, and Uber. How has your journey influenced your perspective on real-time stream data processing?
My journey from MapR, PayPal and Uber covers batch processing, real-time messaging, and real-time data processing platforms. All of them are trying to solve the same type of problem - discovering and creating business value from data. No matter what technology the customer will adopt, their business goal remains the same. Therefore, good technology needs to make it easy for customers to achieve their business goals quickly and in a reliable way. That influenced my perspective on real-time stream data processing. In today's world, how to quickly and easily extract the most valuable information from massive data and apply it to make people’s lives better has become a key consideration of many companies. Real-time data processing is definitely playing a crucial role for these companies to reach their goal.
What are some key areas of interest or innovation in stream processing that you hope to see explored at Flink Forward?
Sure, I’m looking for two key areas of interest. The first being a Simplified usage of stream processing tools, peered with user-friendly interfaces, allowing for a stream processing system that beginners and non-programmers could easily use. Secondly, batch and real-time streaming unification use cases in the industry. I’d like to see more successful batch and real-time streaming unification use cases used in large-scale data lake ingestion and other business areas.
As an Engineering Manager, how do you see Flink Forward contributing to the professional development of your team and the broader community?
Flink Forward provides an awesome knowledge-sharing opportunity for Flink developers from different companies to learn from each other. It helps boost innovations in real-time stream processing and speed up new feature development, maturity, and production adoption. Flink Forward helps both my team and the broader community quickly grow real-time processing professional knowledge and also provides us a good opportunity to contribute back to the continuous maturity of real-time data processing.
What excites you the most about being part of the Program Committee for this year's Flink Forward?
What excites me the most is the chance to actively address the issues and challenges I encountered in real-time data processing, having worked in it firsthand. Having a seat at the table enables me to shape and influence the broader community.
Can you share any insights or best practices from your experience in deploying and managing real-time stream processing platforms at scale?
Having a good observability tool and auto-recovery system is crucial to managing real-time stream processing platforms at scale. Real-time stream processing is usually a long-run application that is required to be highly reliable and resilient to failures. A good observability tool helps detect issues or failures in a timely manner and triggers auto-recovery to keep data processing without data loss. This is a “must-have” for some real-time businesses like Uber. In addition, having a dynamic resource allocation and auto-scaling system to gracefully handle traffic spikes without service disruption is also crucial to managing real-time stream processing platforms at scale.
How does Uber leverage Apache Flink to address specific business challenges or use cases, and what lessons have you learned from these implementations?
Apache Flink is widely used at Uber to support Uber’s various real-time business challenges and use cases. Typical use cases include Uber ride surging pricing support, fraud and security attack detection, Uber driver search and matching for Uber rides, real-time advertisement etc. From these implementations, the top 3 lessons learned were:
- Carefully choose/enable certain Flink features based on the real-business need, i.e., exactly-once processing, checkpointing, etc. There is no single golden configuration suitable for all. Tradeoffs need to be considered to enable certain Flink features or configurations.
- Choose the suitable Apache Flink API to use. For example, some use cases could use Flink SQL without writing complicated Java applications. However, some use cases have to use lower-level Flink APIs to build complicated logic to achieve the business goals.
- Do proper capacity planning and tuning according to the data traffic to avoid job failure.
In your opinion, what are some key factors that contribute to the success of Apache Flink in enterprise environments, and how does Flink Forward support this success?
I think the key factors of making Apache Flink successful in enterprise environments include but are not limited to, highly reliable and highly scalable, simple user interface, ease of operation/maintenance, and a mature ecosystem. Flink Forward promotes innovation in those areas to make Apache Flink more mature and a better fit in the enterprise environment.
As someone deeply involved in the operational aspects of stream processing platforms, what are some common pitfalls or misconceptions that organizations should be aware of?
A common pitfall of operating Flink or other stream processing platforms is not properly allocating resources and/or tuning memory configurations, which leads to job failure or running in an inefficient manner. Another common pitfall of operating stream processing platforms is noisy neighbor issues in a multi-tenancy environment. Without good resource isolation, multi-tenancy could cause unexpected job failure or running in an inefficient manner.
How can companies like Uber contribute to the ongoing development and improvement of Apache Flink, and how does Flink Forward facilitate such collaboration?
Uber engineers aspire to integrate additional new features from Apache Flink, fortify its capabilities, and actively contribute to its enhancement within the open-source community. Recently, my team made some contributions to the Flink native Protocol Buffers Support. As the native Protocol Buffers support gains widespread adoption at Uber, we foresee opportunities to enhance its maturity through our contributions to its development. Through Flink Forward, Uber engineers will have the opportunity to learn more new Flink features and use cases for potential integration with Ube r, which could be potentially adopted at Uber and create opportunities for Uber engineers. Thus, fostering avenues for reciprocal contributions to open-source development. Concurrently, they will also share Uber's Flink use cases and insights, aiding other companies in the adoption of Flink's latest features and use cases.
Looking ahead, what are your expectations for the future of real-time data processing, and how does Apache Flink continue to play a pivotal role in shaping that future?
I’d expect the future of real-time data processing would allow for a powerful and easy-to-plug-in tool that can be used anywhere and by anyone. Similar to iPhone adoption; accessible across various age groups and educational backgrounds . The Apache Flink community serves as a driving force behind the evolution of technology and product direction. This engagement further encourages enterprises to embrace and actively contribute to the development of new features and products. More activity and enthusiasm from enterprises promotes customers to adopt new features and products.
Engineering Manager
Uber
Join the Conversation: Call for Papers (CFP) is now open!
Do you have a great streaming data story to share?
Speaking at Flink Forward Berlin 2024 is a great way to connect with hundreds of your peers, and broaden your knowledge on data streaming.
Be quick, Call For Presentation close 11:59 pm (CEST) on May 17th, 2024.
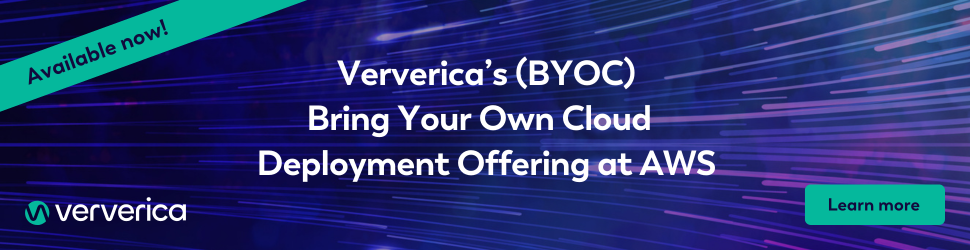
You may also like
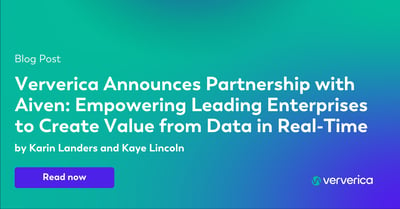
Ververica Announces Partnership with Aiven: Empowering Leading Enterprises to Create Value from Data in Real-Time
Ververica and Aiven partner to unlock real-time streaming data together.
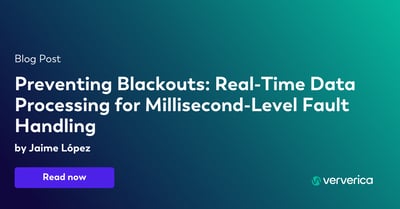
Preventing Blackouts: Real-Time Data Processing for Millisecond-Level Fault Handling
Leverage real-time data processing for instant power outage detection. Im...
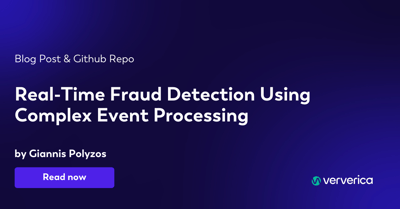
Real-Time Fraud Detection Using Complex Event Processing
Real-time fraud detection with Complex Event Processing helps identify su...
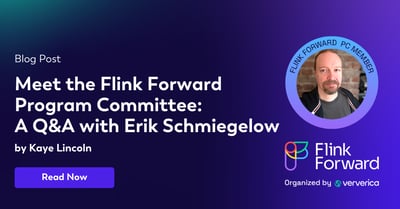
Meet the Flink Forward Program Committee: A Q&A with Erik Schmiegelow
Discover insights from Erik Schmiegelow of Hivemind on the importance of ...